7 Trends and Developments in AI Research from the State of AI 2023 report
This article covers the trends and developments I found most fascinating from the new State of AI 2023 report.
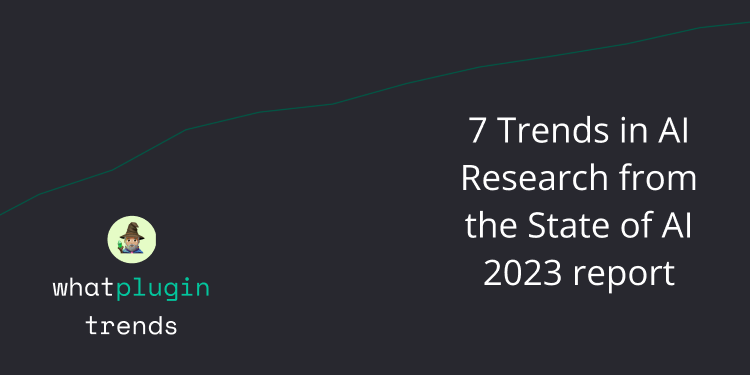
I read through the State of AI report 2023, a 163 slide behemoth of a power point made by AI investors Nathan Benaich and the Air Street Capital team, on a long flight. It's a fascinating read about current and future developments in AI, divided into sections of industry, politics, safety and predictions.
In this article, I've summarised what I think were the most interesting insights from the section on AI research, and broken it down for you to make it more digestible.
Definitely check out the full report if you want more details on each of the points below, or any of the other great sections that the full report covers.
โ
โ
Hereโs the 7 most interesting trends I found on AI research:
โ
1. Closed-source dominates, open-source is trending
- OpenAI and Google have little transparency on their AI models, citing competition and safety precautions (whichever you believe).
- Meta is a counterweight to this with openness on the technical details of their LlaMa models.
- ChatGPT and other closed-source models remain the most popular, but open-source models allowing commercial use, such as LlaMa 2, are trending.
โ
2. Context length growing in importance but has limitations
- Context length, the amount of input language models can process, is increasingly important.
- Anthropic leads the pack when it comes to context length with its Claude model (100k context window).
- However, long context length often donโt live up to expectations. On retrieval tasks, the best models are way more likely to capture text in the beginning or end of a document, while losing out on stuff thatโs in the middle.
โ
3. We could soon be running out of human-generated data
- Limits on human-generated data to train models restricts scaling and makes it challenging to continue the expansion of AI.
- At the current pace, we're likely to run out of high-quality language data by 2026.
- Low-quality language data as well as vision data, are set to last until 2030-2060.
- The problem could potentially be remedied by innovations like OpenAI's Whisper which makes audio data available to LLMs and OCR models like Meta's Nougat.
- Improving quality of synthetic data (ie training LLMs on their own outputs) also shows promising results and could help break the โdata ceilingโ.
โ
4. Developers relying on "vibes" over benchmarks for choosing LLMs
- AI developers generally seem to disregard benchmarks like Standford's HELM and Hugging Face's LLM leaderboard when deciding which models to use - and rather trust their vibes.
โ
5. Prompting getting sophisticated and automated
- The process of improving prompts to boost performance of LLMs is getting more sophisticated.
- Chain of Thought prompting (asking for intermediate reasoning steps) and Tree of Thought (representing โthoughtsโ as nodes in a tree structure) are becoming popular techniques.
- LLMs themselves are apparently great at prompt engineering, evident by results from new tools for automatic prompting (such as APE and OPRO).
- A current challenge, particularly for app development using GPT-4, is changes to the model making it difficult to get stable results from the same prompt over time.
โ
6. Chatbots integrating with image generators for user-friendliness
- Co-pilot style interfaces for guiding image generation and editing is trending. Apps are implementing instruction based assistance that makes it easier for users to iterate on their images.
- DALL-E 3 inside ChatGPT is the most recent example of this. Itโs now possible to tell DALL-E to make adjustments to the image inside the chat.
โ
7. LLMs making advances in life sciences
- LLMs are paving the way for advances in science that were previously out of reach.
- Of all scientific fields, Medicine has the highest increase of studies of applied AI in the last year.
- Highlights of new research using LLMs include: designing novel proteins, making prediction of protein structure faster and cheaper, understanding gene perturbations at scale and without the need for cell-based experiments and predicting if changes in amino acid changes are benign or pathogenic.
- Answers to consumer medical questions generated by Google's Med-PaLM 2 model was preferred over physician answers in most areas (evaluated by physicians). Google has recently been adding multimodal capabilities that go beyond text-based Q&A.
- Accuracy is crucial in the medical field and AI is still not perfect; dedicated models for helping decide whether to rely on AI models or reverting to a clinical workflow show promising initial results.
โ
If you want to delve deeper, check out the State of AI report which in addition to AI research also covers industry, politics, safety and predictions.
โ
โ
โ
โ